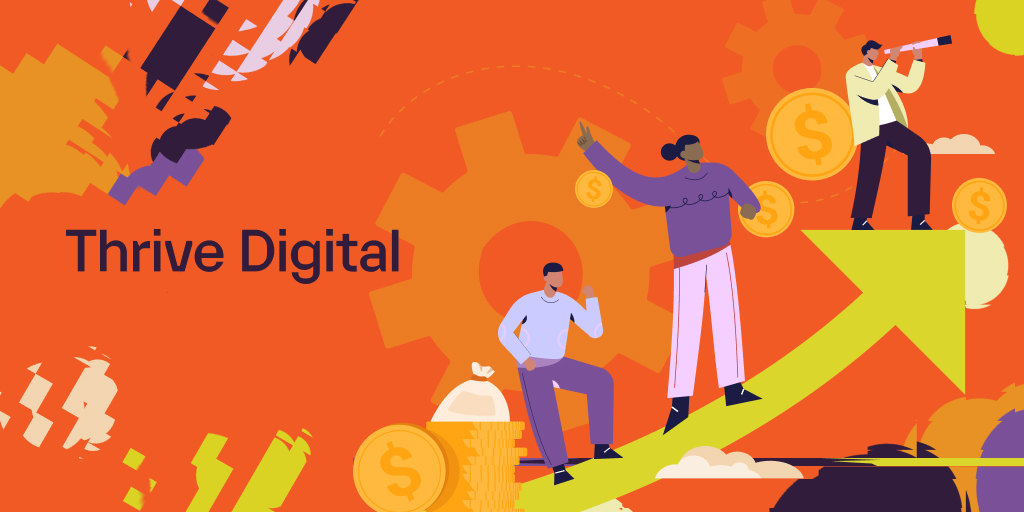
TL;DR: Geo experiments can show us the real sales impact of ad campaigns but should be designed and interpreted carefully. These experiments assess how advertising influences sales without compromising privacy, dividing regions into test and control groups. It's crucial to consider regional variables, historical data shifts, and test duration when designing experiments — validating results through multiple tests is essential. This form of measurement can help us with over-reliance on cookie-based attribution.
Geo experiments are a powerful way to better understand the incremental impact of your advertising program on sales. They are privacy safe and relatively easy to execute with modern digital ad campaigns. They’re not perfect though. They can sometimes be difficult to design or get a clean final result that can help you make decisions about your marketing program.
Let’s explore how to run a successful geo experiment, what to look out for and where to find additional information.
What are geo experiments?
Geo experiments are an experimental design used to evaluate the incremental impact of marketing efforts on a key business metric.
Different geographies are split into two groups:
A) A test group where some marketing change is introduced - for example, a new TikTok advertising campaign.
B) A control group used as a baseline for what would happen during normal, business-as-usual activity.
Results are calculated by analyzing the relationship between the holdout and test groups historically. This relationship is used to create a counterfactual for the test group - a prediction of what might have happened if no change was made in the test group.
Geo experiments are conducted on TOTAL sales rather than using any attribution model to assign value. what is measured is the incremental impact to total sales instead of relying on additional software.
Are geo experiments privacy safe?
Geo experiments are privacy-safe as they do not require cookies or personal information to run.
Additionally, geo experiments do not require installing any third-party software or tracking pixels on your website and the only required information to run an experiment is the total daily sales by geo.
What can geo experiments measure?
Geo experiments are typically used to measure the incremental impact to key business KPIs like sales or high intent signups but can also be used to measure the impact to other metrics like organic and direct traffic.
The key point here is that geo experiments measure only the conversions which wouldn't have happened without advertising. This is the true impact from your ads and is what we refer to as incremental conversions.
Note: Geo experiments aren’t great at measuring long-term effects from marketing or advertising, so brand awareness campaigns and other tactics meant to drive value over a long period are difficult to measure with geo experiments.
Most types of brand campaigns also have a short-term effect measurable by geo experiments but the longer-term effects aren’t easily captured in the results of these experiments.
Major considerations in designing a geo experiment
There are a few major considerations when designing a geo experiment critical to getting an actionable result.
Consider confounding factors
The first is to consider any confounding factors that may impact sales in the test region. Because these experiments look at total sales and not attributed sales, confounding factors can make a test result look better or worse if they’re not controlled for.
Examples of confounding factors include:
- Promotional periods differing across different geographic regions
- Seasonality being different in different geographic regions
- Offline factors and merchandising
Consider historical context changes
The second major consideration is your historical data. If there were major changes to the relationship between the test and control groups historically, there may be changes to this relationship during the test period.
It’s important not to disregard these past changes to the relationship between the two groups and simply choose a shorter historical period to increase the model fit. This can lead to incorrect estimates of confidence or credible intervals in the final result, and give you more confidence in results than makes sense.
Consider the minimum detectable effect
A third major consideration to designing a geo experiment is the minimum detectable effect that is measurable in your test design - and most importantly, is it feasible?
In order to get a clear test result, the change to your marketing program being tested needs to overcome the noise in your KPI data.
Here is a basic example for a test design with an estimated 5% minimum detectable effect testing a new Meta Ads campaign:
Consider the length of the test
Every marketer wants to test things quickly but geo experiments should be given enough time to understand the impacts you're trying to measure.
Channels such as Branded Search will have much more immediate effects and can be measured in a shorter period.
Channels further up the funnel, however, may take longer to start seeing an effect as consumers first need to consider making a purchase before they actually do. In these cases, your test length may have to be 2 or 3 times longer than the consideration cycle of the product you're selling.
Interpreting results from a geo experiment
One of the more difficult aspects of running geo experiments is the uncertainty that comes along with the output.
With any experiment, there are two primary types of errors:
- False positive - the test shows the experiment had an impact on sales but there was no “true” impact
- False negative - the test doesn’t show that the experiment had an impact on sales but there was an impact
Along with these types of errors, a well-run test will also estimate the range of possible “true” outcomes of the test. The underlying interpretation of these estimates is beyond the scope of this article but interpreting this range of results can be difficult in certain situations.
Take for example a test where the range of outcomes includes both profitable and not-profitable outcomes. The test result can show there was an incremental impact from your advertising but you still don’t know whether that impact was truly profitable for the business or not.
This is where test validation comes in. We interpret any one geo experiment as a single data point from a single point in time. To get a robust picture of the impact a channel or strategy is driving, multiple tests must be run in different seasons and at different spend levels. Testing this way will not only help you to validate the results of the first test, but it will also make decision-making much easier as there will be multiple data points to evaluate.
Building geo experiments into your measurement strategy
Bringing everything together raises some critical questions:
- How often should we be testing?
- How do we integrate the test results into decision-making?
How often should we be testing?
We think organizations should be testing as often as is feasible for their marketing program. This means that organizations investing less into paid media every month will be testing less often than organizations investing millions of dollars.
One basic framework is to follow these general steps:
- Test all new channels when they are first launched and again 3-6 months after launch. Ideally, this is done at different spend levels to understand the rate of diminishing return.
- Test the top 1-2 channels in your advertising program at least twice per year during different seasons or spend levels.
- Test low-incrementality channels like Branded Search at least once per year.
How do we integrate the test results into decision-making?
We encourage marketing teams to think of tests as building evidence. A single test provides a single data point and is therefore unreliable to be applied across the entire year or at different spend levels. As you run additional tests for a given channel, the evidence grows and you can be more confident in the dynamics of that channel.
Additionally, the more time that passes after a test is run, the less reliable it result becomes. This is because the performance of channels can be impacted by several factors including seasonality, platform optimizations, market factors, and more. Keep this in mind when making decisions based on test results.
We also recommend considering the dynamics of diminishing returns when using test results. Just because a campaign produced a profitable incremental result at a certain spend level, doesn’t mean increasing the spend will produce the same efficiency - it almost certainly won't. This is another reason why testing a channel at multiple spend levels can drive a better understanding of the dynamics needed to make better investment decisions.
Finally, be cautious when associating geo experiment results with cookie-based attribution. It is fairly common for marketing teams to create multipliers that tie an incrementality result with the results seen in an ads platform (like Meta Ads). The problem is that an increase in performance from a given ad campaign doesn’t necessarily mean the incremental impact is also increasing. We recommend cookie-based attribution only be used to measure the relative performance in a campaign that has been tested and not used as an indicator of incremental performance.
What now?
Geo experiments are a powerful tool to understand the true impact of digital advertising on business outcomes - they can help you to measure incrementality, and to make better media investment decisions that drive sales.
However, geo experiments aren’t a silver bullet. They can be difficult to design and difficult to interpret. One of the best ways to tackle these challenges is to gradually build a testing program that regularly prioritizes and executes geo experiments across your marketing program. This will build internal expertise, generate strong evidence to make investment decisions, and put incrementality at the forefront of your marketing team’s incentives. And if that doesn't work, just call us.
Additional Reading
https://research.google/pubs/measuring-ad-effectiveness-using-geo-experiments/