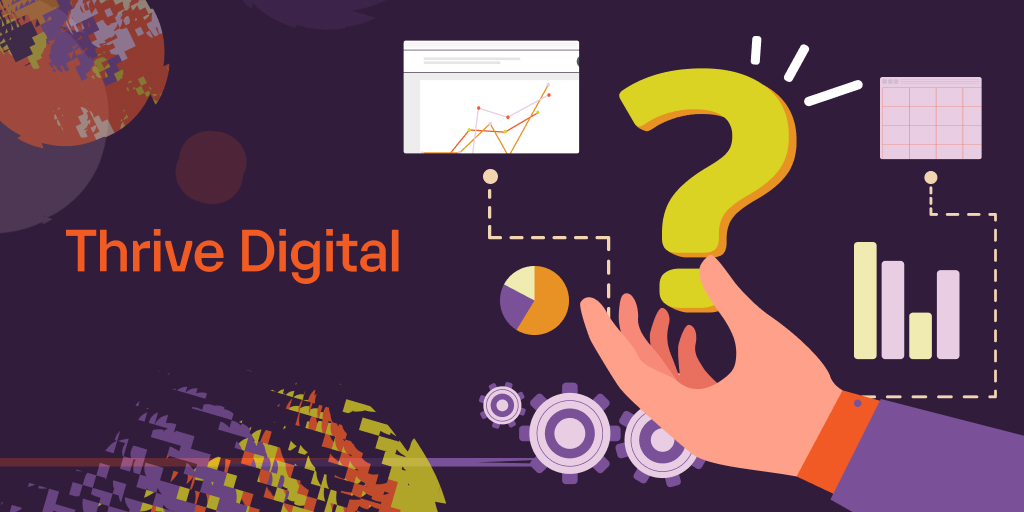
We juggle a lot as growth marketers. Different tools, platforms, campaign types, strategies, creative options, and business objectives — just to name a few. But for all the testing we do to stay ahead of the curve, how do we test for the effectiveness of what we choose to focus on? Enter hypothesis testing.
I sat down to chat with our Associate Director of Strategy, Madeline Silver, to talk about this topic and we really got into the weeds. If you’re curious about how to understand the impacts of your campaign with more confidence, this interview is for you.
Santana Blanchette: Hi Madeline! Glad you could sit down and chat with me today about hypothesis testing. Let’s start with the basics — how would you define a hypothesis in advertising and why are they important?
Madeline Silver: If we can remember from our early days in grade school, a hypothesis is just a statement about what we think is going to happen when we test a question. Something that can be proven or disproven. For example, in advertising if the question is, “Is this channel profitable for us?” our hypothesis might be, “We believe this channel is profitable above our 200% incremental ROAS target.”
I won’t get into the technicals of p-values or null hypothesis here, more simply it’s just anything we’re trying to get answers to prove or disprove our beliefs about.
How are hypothesis tests used in strategic decision-making for marketing teams?
Madeline: All decision-making at this point in the digital advertising industry is heavily underpinned by data — we just have so much available. But we’re also more aware now with changes to the privacy landscape like the way platforms track and collect data, having multiple sources of truth, and just understanding how messy things are in actuality that we can’t just take single data points as ground truths to explain cause and effect. Or what works and what doesn’t. There's a lot more complexity there.
So for example, if you launched a new channel and you saw a 200% increase in revenue, can we say with certainty the increase in revenue was due to the changes we made with the new campaign? Or was it due to a change in seasonality, something a competitor started doing, or a change to the product or website funnel? Using hypothesis testing and experimental frameworks enables us to ask questions and build confidence in our ability to answer those questions because we’re able to isolate our variables, control for the inherent noise, and cut through a lot of that fat.
What is a common misconception about performance and hypothesis testing?
Madeline: There are a lot of misconceptions or misunderstandings. One metric in general that can be confusing for marketers is statistical significance. This metric is typically important when you’re running any kind of experiment, A/B test, or lift test. For example, if we were running an A/B test or a lift test and got a 20% improvement to our KPI and a statistical significance of 90%, that’s a strong statistical significance or confidence. But a lot of people will misinterpret it as confidence in our effect. So they’ll say, “We got a 20% increase which means we have a 232 ROAS and we’re confident that is our result.” That’s not actually what statistical significance is telling us. There’s still an expected range of value in the result we could have gotten. Rather, statistical significance is saying we’re 90% confident the effect we’re reading occurred because of the change we made in this experiment and not because it could have happened by chance.
On the opposite side, you could have a hugely dramatic result. For example, Creative A drove 300% higher ROAS than Creative B at the same cost. It looks like Creative A is the better bet but your statistical significance for Creative A could have been something like 60% which is only a little bit better than a coin flip. What that’s telling us is that if we were to run this test again, we only have a 60% chance that Creative A would come out the winner again. Does that mean we should only move forward with Creative A? Probably not because Creative B could win 40% of the time.
With experiments, it’s important not to get too focused on the false granularity of, “this is the answer”. And not to misinterpret statistical significance as confidence in that answer but really thinking more in terms of probabilities. “We feel confident this is the stronger answer with this amount of certainty and we want to move forward in this way” rather than, “This is the answer down to a decimal point.”
What is statistical significance?
If you’re a less technical marketer like me, you’re probably wondering by this point in the interview, “What the heck is statistical significance?”
I had the same question so I turned to our in-house stats experts and they gave me this helpful definition:
Statistical significance (oversimplified here for lay person's purposes) is the likeliness that the effect seen in an A/B test is due to random chance, or if it is a result of the treatment of the test. When something is statistically significant, it typically means there is less than a 5% chance that there is no real difference between the treatment (B) and control (A) in a test.
What is an underutilized tactic or method for hypothesis testing?
Madeline: Validation and retesting. An experiment is superior in that you have controlled for your other variables and there is a very good set-up but I think we can get overconfident in attaching ourselves to a single answer. An experiment is still only a point-in-time answer, we’re only going to be running it for a few weeks, a month, a year, to answer a specific question. You could run that same experiment in a different season and get a different answer or there could be so many other variables that impacted the scale of the answer you got within the test based on that point and time.
Let’s look at an example using channel profitability. It’s a single data point and it’s directional. Let’s say we look at this data point and it tells us we can confidently say this channel falls below our desired profitability. Now based on the other context, this channel might be 60% of your ad spend. That doesn’t necessarily mean we take the single data point and go and pause it all. Rather, we recognize we have one data point. Let’s look into what might have caused the result or how we could slightly adjust and revalidate again to get slightly more confident as marketers that this data point aligns with other data points and directionally allows us to make these bigger decisions.
Another example would be if you’re running an A/B test to a landing page and you put 20% more traffic to variant B and you get a winner so you switch it over. Are you running a test to revalidate the winner against that previous control? Because if you just redid the test but the opposite, would you get the same results? I think we can get overconfident in relying on single data points and in false granularity. We should be thinking more in ranges and probabilities.
Are there any ways that marketers can easily improve their testing?
Madeline: Yes, there are lots of ways. I’ll start by giving you the stock marketer's answer, “To get better at testing you need to understand your objective.” I think that’s the number one thing any marketer would tell you because it is true. You need to understand the question you’re asking. Also, what variables you are actually measuring and comparing against each other to support or reject your hypothesis?
For example, I’ve seen a lot of occasions when a marketer thinks they’re testing A vs B when really they’re testing A vs B plus C plus D. That happens a lot nowadays when we have these new campaign types or strategies like Performance Max or Advantage Plus or even broad match keywords. If you’re going to go test broad match keywords, does it make sense to test broad match against exact match? No, because that’s apples to oranges. You should be testing introducing broad match to your existing structure which is maybe an exact DSA phrase match. You’re testing one system against another and you need to make sure that is apples to apples.
Another example is spend. Even outside of a proper experimental set-up. Let’s say you launch two creatives and you see one has a much higher ROAS than another but it could have 1/10 of the spend. We’re not factoring in the impact of diminishing returns and scale into our assessment of A vs B. Is that a valid way to say one has a stronger ROAS and is more successful? No. Again, it’s apples to oranges.
I think there are a lot of opportunities for us to get more defined and strategic with the way we set ourselves up with our questions and look at our variables with a more critical lens.